Generative AI: And the winner is...
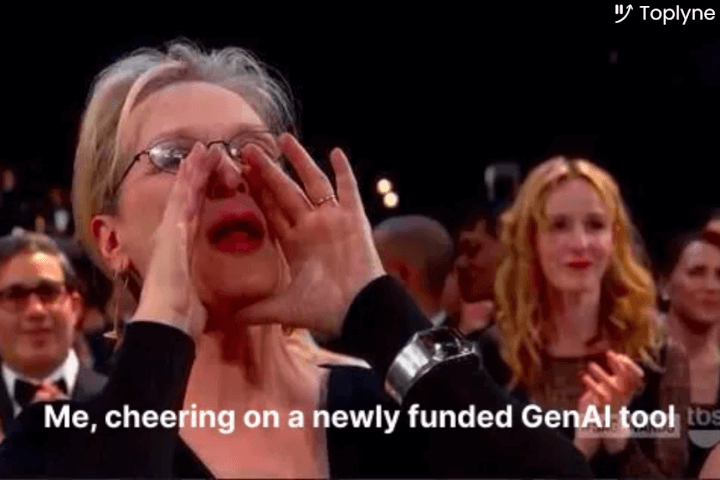
“What I cannot create, I do not understand.”
The last words etched on Richard Feynman’s blackboard as he succumbed to cancer in the winter of 1988. Feynman’s final injunction to his students and to the world. (Good luck topping Feynman quotes, ChatGPT)

Feynman’s last words are, among other raving adjectives, timely in 2023.
You can look far and wide, but you’d be hard pressed to find a more succinct TL;DR of the biggest gold rush of our times: Generative Artificial Intelligence.
OpenAI alludes to the same Feynman quote:
“Generative models have a number of parameters much smaller than the amount of data they are trained on, so the models are forced to internalize the essence of the data in order to then generate it.”
In other words, for these models to create, they must first understand.
Since we released part 1 of this series on Generative AI just last week, we saw two major announcements happen.
- Google announced Bard (the same LaMDA that Blake Lemoine was convinced was sentient) and…
- Microsoft teased a chatbot UI for Bing… which was reviewed by the New York Times as “a moody, manic-depressive teenager who has been trapped, against its will, inside a second-rate search engine.” and by Ben Thompson of Stratechery as “…the most surprising and mind-blowing computer experience of my life.” So
- …mixed.
We expect fresher drops and hot-takes this week. This is just the nature of Cambrian explosions.
For scale and reference, just look at the timeline of events in text generation ever since GPT-3 ran the four-minute mile:
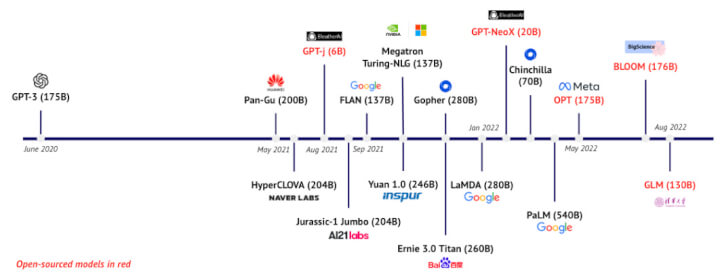
These are uncharted waters, and we’re learning the rules of the game on the go.
At least some of the rules.
Across the Generative AI stack, there are four key levels we’ve seen emerge:
- The silicon - The Nvidias and Tensors of the world. The shovel sellers in the gold rush.
- The cloud - The AWS, GCP and Azures of the world that expose the silicon to developers.
- The gold - Open and closed-source models like GPT-3, Stable Diffusion.
- The magic - Apps that expose the magic to the average B2B or B2C user. Think Jasper, Copy.ai, Writesonic, or Murf.
How GenAI will scale the hitherto human-controlled spheres of content-creation is less predictable, and arguably anyone’s guess at this point.
But at the application layer, where creation has meant that of real market cap and value, and not just hype, the outcomes are arguably more predictable.
Because here, the rules of the “old” world of SaaS and Product-Led Growth (largely) still apply.
Who wins?🏆
So far, we’ve seen the emergence of 350+ companies in this space that have raised a combined $7B+. Many have already reached $100M+ in annualized revenue. But it’s still early days in this race, and too early to call a clear winner.
But if you’re one of the 350+ companies in this space, you’re playing largely the same game that we’ve seen play out in many emerging SaaS categories before. With some additional color of course. Our God-given neural networks have understood these rules well enough to make some reasonable predictions.

In analogous markets, the winners of hype cycles and emerging markets have historically been companies that have been able to:
- Drive data network-effects
- Differentiate strongly
- Embed themselves in existing workflows of users
- Hyperpersonalize their GTM
- … and scale enterprise
Here’s what that might look like for the application layer in Generative AI…
Network effects, but with data 🔁
Typically data network effects kick in when, as more users start using your product, the data from their usage feeds back into your product positively such that adding each incremental user also makes your product better in some sense. Classic virtuous cycle traits.
Driving data-network-effects at the GenAI application layer will likely be done in two ways:
- By sculpting a niche - by building fine-tuned models on top of foundational models for particular verticals using proprietary data.
- …or
- Going end-to-end - Most GenAI companies today interact with the model layer through APIs, and do not own their models. But if the core driver of value is the model itself, many may have to verticalize.
Herd purple cows 🐮
(Or drive strong differentiation)
This is a space that’s currently dominated by a few first-movers and many copycats all competing on the merits of largely hype and distribution. While the incremental merits of product performance may be real, they’re currently too limited to drive any real differentiation.

How do you drive differentiation and stand out in such a space? Some have done it well:
- Integrations - Jasper pulled away in the text-generation space by driving differentiation through the integrations on offer: Jasper was the first to integrate with the darlings of every content-marketer: SurferSEO, Grammarly, and Copyscape
- Interaction layer - All GenAI tools need is a text box for an input. Those that are able to innovate with the nature of the interaction layer to drive differentiation have a first-mover advantage with distribution. Midjourney’s interaction layer is Discord, while GitHub Copilot is now generating nearly 40% of code in the projects where it is installed.
- Narrow positioning - By driving their messaging to hyper-specific use cases and personas, rather than casting a wide net. Think Gong in conversational intelligence.
- Foster communities - Growing your own communities centered around your product is a powerful weapon to drive advocacy, word-of-mouth, referrals, and feedback loops.
Embed themselves in existing workflows of users 📌
As the top of the funnel of the application layer blew up, so did the one-and-done users dropping by for casual exploration. During these hype cycles, these products can only hold user attention for so long.
Unless they’re able to attach themselves to these users’ existing workflows and behaviors, rather than attempt to train new patterns of usage and behavior.
Take Jasper again, for example, who launched a Chrome extension in October, 2022 - Post launching the Jasper Chrome Extension, Jasper stopped sharing attention with every other tool in content workflows. The AI sidekick became ubiquitous - because its new home was Chrome.
Hyperpersonalized GTM 🦾
Generative AI has found its way even to family groups and dinner conversations. That’s when you know that your top-of-funnel has exploded. The application layer is now attracting wide swathes of usage.
Across different:
- Sources - Across search, social media, press, events, etc. - sure, this is common. But one level deeper, specific influencers with niche audiences are driving a lot of traffic to these tools. For eg:- Pictory started growing 50% MoM after a post that went viral about how ChatGPT could be used along with Pictory to grow your YouTube channel.
- Levels of intent - Within the noise at the top of the funnel, there are tourists, champions, aficionado, explorers, and even the occassional enterprise buyer.
- Personas - Within B2B audiences, anyone who has to generate content as part of their job has a use-case with the application layer. And that’s just about anyone from sales, marketing, customer-success, product, rev-ops, developers - wait, we’ll save you some time - that’s just about anyone.
And while top-of-the-funnel growth has been staggering, it has failed to trickle further down the funnel into revenue growth. Monetization isn’t a figured out science for these tools just yet.
One way to approach GTM is to be broad-brush and use a one-size fits all approach for your freemium user-base in an attempt to drive your revenue motions: conversion, expansion, and churn reduction.
But if we’ve learned anything from SaaS’s shift to product-led growth, it is that GTM wins on hyper-personalization. And the what, when, where, and how - of all your marketing, sales, and sales-assist touches need to feed off of product-usage, firmographics, and demographics to drive your monetization engine.
With the amount of noise at the ToFu of GenAI companies, those who win at GTM will be those that win at product-led engagement.
Related side-note: Hyper-personalizing your in-app engagement is one way to drive conversion and retention. Check out how the GenAI tool Pictory drove their NRR up by 14% using Toplyne.
Scale enterprise 📈
How long do you reckon, until James Cameron gets Hollywood to embrace generative AI and production houses throng to release the first fully artificially generated movie of all time? At this point, it’s a safe bet to say that it’s… inevitable.

As foundational models become open-sourced, and the model costs hit the floor, generative tech will likely become almost universal. At that point…
“The actual value in AI shakes out in kind of the white glove service for the biggest content providers, like Disney and Sony,” says Jim O’Shaughnessy, executive chair of Stability.AI (speaking to Evan Armstrong)
Scaling enterprise will require the application layer to adapt to custom workflows, build white-glove support, stay nimble to customizations, security features that protect the IP of large content houses, and evolve GTM.
Watch out for…
350+. Keep an eye on that number. With barriers to entry fully levelled by no-code product-led growth tools in the last ten years, you can launch a product today from the comfort of your living room.
But they all compete on end-points that we’ve come to understand better in that time.
To repurpose the Feynman quote, the value they create, we understand (to a large extent.)
In Part 1 of our deep-dive on Generative AI, we covered how we’ve gotten to the point we’re at today - the inflection points that have led to this gold rush. Look out for Part 3 in your inbox next week.
That is unless Bing Chat goes rogue and wipes out all of civilization as we know it.