Predictive Lead Scoring for PLG + A Tool For It

Product-led growth (PLG) is a growth strategy where the product is the driver of customer acquisition, engagement, retention, and expansion. A PLG company creates a valuable and user-friendly product that customers can try for free as part of a limited period free trial or a freemium product that makes basic features available for free and charges for premium ones.
With the product being easy to adopt, there is much less intervention required from sales and marketing teams. If customers get the outcomes they desire from the product, there is a very high potential they will become loyal users and refer the product to others. This lowers customer acquisition costs, takes the weight of sales and marketing teams, and establishes a growth loop, advantages that are no doubt attractive to businesses.
How does it translate to real-world business benefits? While product-led companies do outperform their sales-led peers in revenue growth, valuations, and operating efficiency, most companies may not necessarily receive the fruits of a PLG motion, reveals McKinsey research of B2B SaaS companies. A hybrid of product and sales, aka product-led sales, is the better bet. For more perspective, head to this article.
Define predictive lead scoring and its critical role in enhancing PLG strategies.
- The PLG motion focuses on creating the best user experience and letting the product and service (self-serve) speak for themselves. But first, you must find the right potential leads for your product, which means your sales team must do a fantastic job of prioritizing your best leads. Even with demographic and purchase data and good judgment, some leads are bound to fall through the cracks simply because this traditional approach is subjective and inefficient.
- Enter predictive lead scoring, which automates the process for your sales reps so that they can focus on selling. Predictive lead scoring is an algorithm-based machine learning model that uses various types of customer data to calculate a score for each lead. It's accurate and fast, supporting your PLG strategy and saving your salespersons several hours every sales cycle.
Understanding Predictive Lead Scoring in the Context of PLG
Predictive lead scoring is highly data-driven. It uses the following types of data to score leads:
- Demographic data (primary customer data like names, ages, DOBs, and addresses)
- Billing data (how often do customers buy your products and which ones they choose)
- Engagement data (website views, link clicks, freemium downloads, and more)
- Company information (your potential customer's industry, company size, revenue, and more)
- Campaign data (performance of your sales and marketing campaigns to understand future customer behavior)
- Product-usage data (how users interact with product features and actions they take)
A predictive lead scoring model is different from traditional methods in that it is automated and uses multiple data points on users for a well-rounded assessment.
Let's take a quick look at how predictive lead scoring works:
- Identify the data point and target attribute you want to measure.
- Specify a value for each data point. The predictive lead scoring model uses machine learning algorithms to create a model that accurately calculates a lead's score based on their demographic and historical data.
- There are multiple approaches to assigning an accurate lead score. The most common machine learning model, logistic regression, uses a statistical approach to estimate the probability that a lead will convert.
Discuss the importance of predictive lead scoring in identifying high-potential users and optimizing conversion rates in a PLG model.
Winning at PLG is about finding users willing to buy your product. Great sales tactics aren't a fallback in this growth strategy, and even if they were, how do you convince someone to be a customer when they aren't ready?
Predictive lead scoring makes life simpler. Intelligent algorithms use customer data from your CRM, user database, and analytics tools to score each lead quickly and accurately, severely reducing the human error prevalent in the traditional method and optimizing your conversion rate. You save a ton of time and create better alignment between your sales and marketing teams with one tool rather than separate processes.
The Benefits of Predictive Lead Scoring in PLG
Enhanced targeting: Focusing on leads most likely to convert.
With prioritized leads, your sales reps can spend more time on leads that are likely to convert. As more data accumulates in your systems, prediction quality increases, further driving up sales.
Improved resource allocation: Directing sales bandwidth more effectively.
In a scenario of poor lead prioritization and weak sales, your sales leader may want more hands on deck to chase leads and meet targets. Or they may have difficulty assigning experienced sales reps to the most promising leads or potentially large deal wins. With lead scoring done accurately and rapidly for you, resource allocation becomes simpler and need not go through iterations.
Increased conversion rates: Tailoring interactions based on predictive usage insights
Behavioral analysis is built into a PLG predictive lead scoring model. For example, you can leverage user and usage insights to craft targeted messages that persuade users to move to a paid subscription.
Challenges in Implementing Predictive Lead Scoring for PLG
As a predictive lead scoring system relies on data, you need a way to collect user data from touchpoints, such as email engagement, social media interactions, website visits, in-app behaviors, and more.
Building a good predictive lead scoring model without large and high-quality datasets is difficult. When done manually, gathering firmographic data, buying preferences, behavioral data, and other data points is time and effort-intensive. Moreover, the predictive accuracy heavily depends on the quality of data fed to algorithms.
A machine learning model may predict lead conversion in different ways: logistic regression, decision trees, or neural networks. Of these, logistic regression is more popular, given its ease of use and the interpretability of model parameters.
Toplyne: Predictive AI for Sales and Marketing teams
Here’s how companies like RocketReach, Murf, and Vercel generate sales pipeline from their self-serve funnel using Toplyne:
- Step 1/7: Create monetization playbooks to surface conversion and expansion opportunities (leads most likely to convert to paying customers, and teams most likely to grow into larger teams)
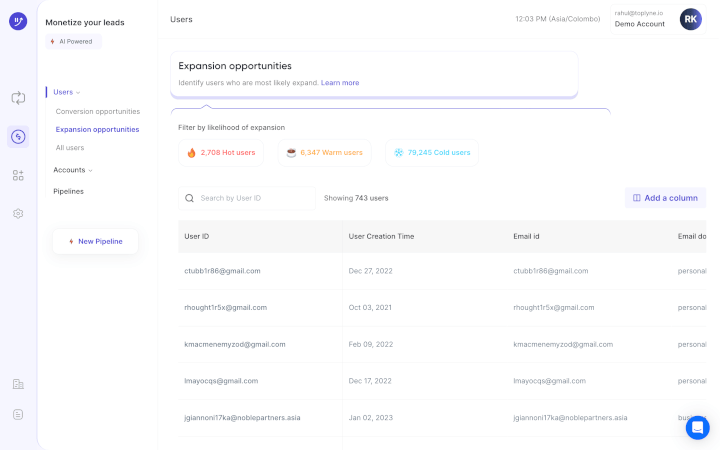
- Step 2/7: Choose the right leads to target – users (individual users) or accounts (a group of users with an organization).
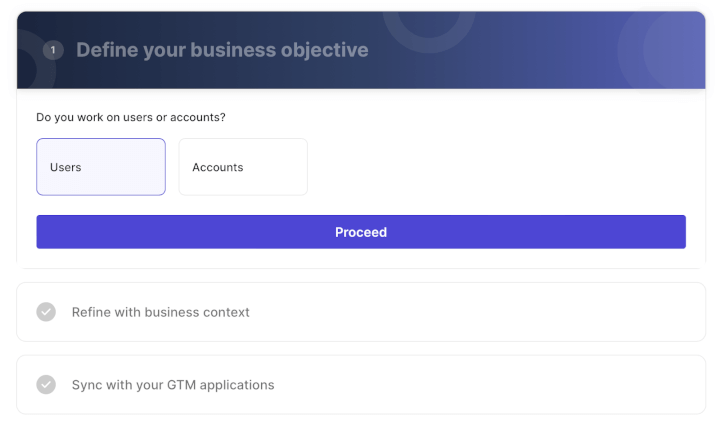
- Step 3/7: Select the frequency at which you would want leads synced in your GTM apps.

- Step 4/7: Define how many leads you want by either the number of leads or your expected win rate, depending on your sales capacity and GTM strategy.
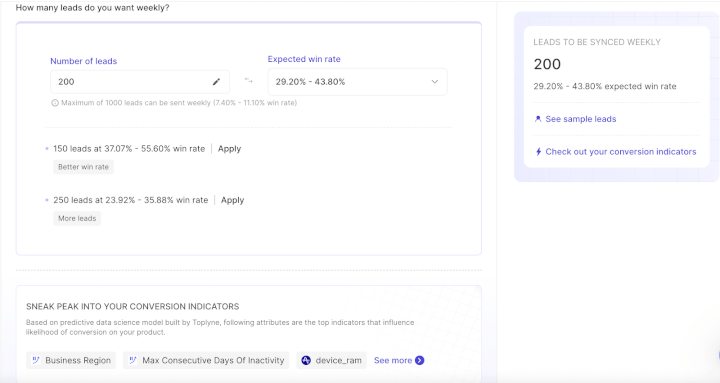
- Step 5/7: Build custom segments - Build custom segments based on And/Or logic at the deepest level of sub-properties within your product analytics.

- Step 6/7: Validate your GTM strategy - Hold back some users as a control group to test your GTM strategy.
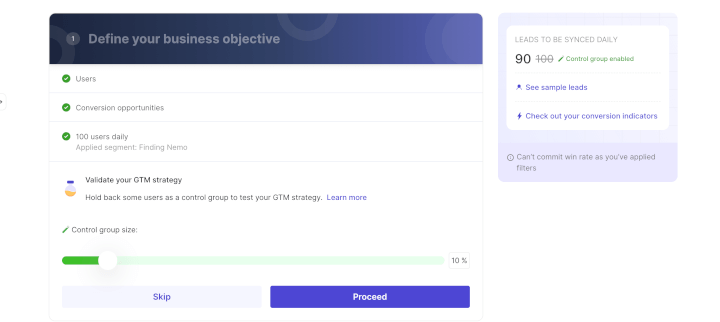
- Step 7/7: Sync your product qualified pipeline into your GTM destinations - CRMs, sales & marketing execution tools, and customer engagement platforms.

Synthetic speech company Murf lets users generate “human-like” voiceovers without buying recording equipment or hiring a voice artist.
Its free tier allows users up to 10 mins of voice generation and transcription. This provides enough value from the product for users to upgrade to a paid plan.
Murf saw a 7x lift in its free-to-paid conversions by using Toplyne to surface conversion pipeline in Brevo, where their sales teams trigger email sequences prompting a discount code to upgrade to a pro plan.
Conclusion
PLG companies today sit on a goldmine of first party data - across product usage, billing, and CRM - that sales and marketing teams have limited access to. Product analytics tools are built for product teams, enrichment tools are built for Ops teams, and billing tools are built for finance teams. Sales and marketing teams are left with no way to use this data to drive metrics they care about.
Toplyne consolidates customer data across product analytics, CRM, and billing tools , combines it with third-party data sources, and pairs this data with custom predictive AI models to accelerate top-line results for sales and marketing teams in SaaS companies. See Toplyne in action and get started on predictive lead scoring for your PLG company.