Why syncing your Google Analytics data to Big Query is a no brainer
Why syncing your Google Analytics data to Big Query is a no brainer
Why syncing your Google Analytics data to Big Query is a no brainer
Here are the top reasons why syncing GA4 to Big Query is a no brainer
Here are the top reasons why syncing GA4 to Big Query is a no brainer
Here are the top reasons why syncing GA4 to Big Query is a no brainer



In December 2023, Google announced the next step in phasing out cookies globally. Google started testing Tracking Protection on Chrome, a new feature that limits cross-site tracking - signifying another big stride towards the end of the cookie-era. This comes on the back of Apple severely limiting advertiser access to IDFA, all indicating a general trend of third-party data's slow march to irrelevance.
As marketing teams scurry away from third party data to build audiences and targeting lists, first party data sources - the Google Analytics, Mixpanels, Amplitudes of the world are steadily moving to the center stage.
And for these marketing teams, Google Analytics is a treasure trove of data on user-behavior - both historical and current. As this data becomes a crucial cog in a marketer's segmentation arsenal, access to historical GA data is table-stakes.
And very few teams even own their own data for the simple reason that GA doesn't support storage of historical data.
Unless you've synced to Big Query.
Here are the top reasons why this is a smart move ➝
1. Data Retention
Data retention refers to how long a platform keeps the data before it's automatically deleted.
While Google Analytics 4 gives you the ability to set the amount of time before user-level and event-level data is automatically deleted from it's servers, there is a limit to the maximum length of time you can set for your data to be retained.
GA4 Limitation: In GA4, user-level and event-level data retention is limited to a maximum of 14 months. With the default data retention time set at 2 months. Please change this to 14 months if you haven't already.
BigQuery Advantage: BigQuery is a cloud based data warehouse and it's first job is to allow indefinite data retention, which means you can store your analytics data for as long as you need without the risk of deletion.
What this means for you: With timeless data retention in Big Query, you have access to all historical data, enabling thorough trend analysis over long term periods and the flexibility to conduct year-over-year comparisons without data loss. This depth of insight supports more informed strategic decisions.
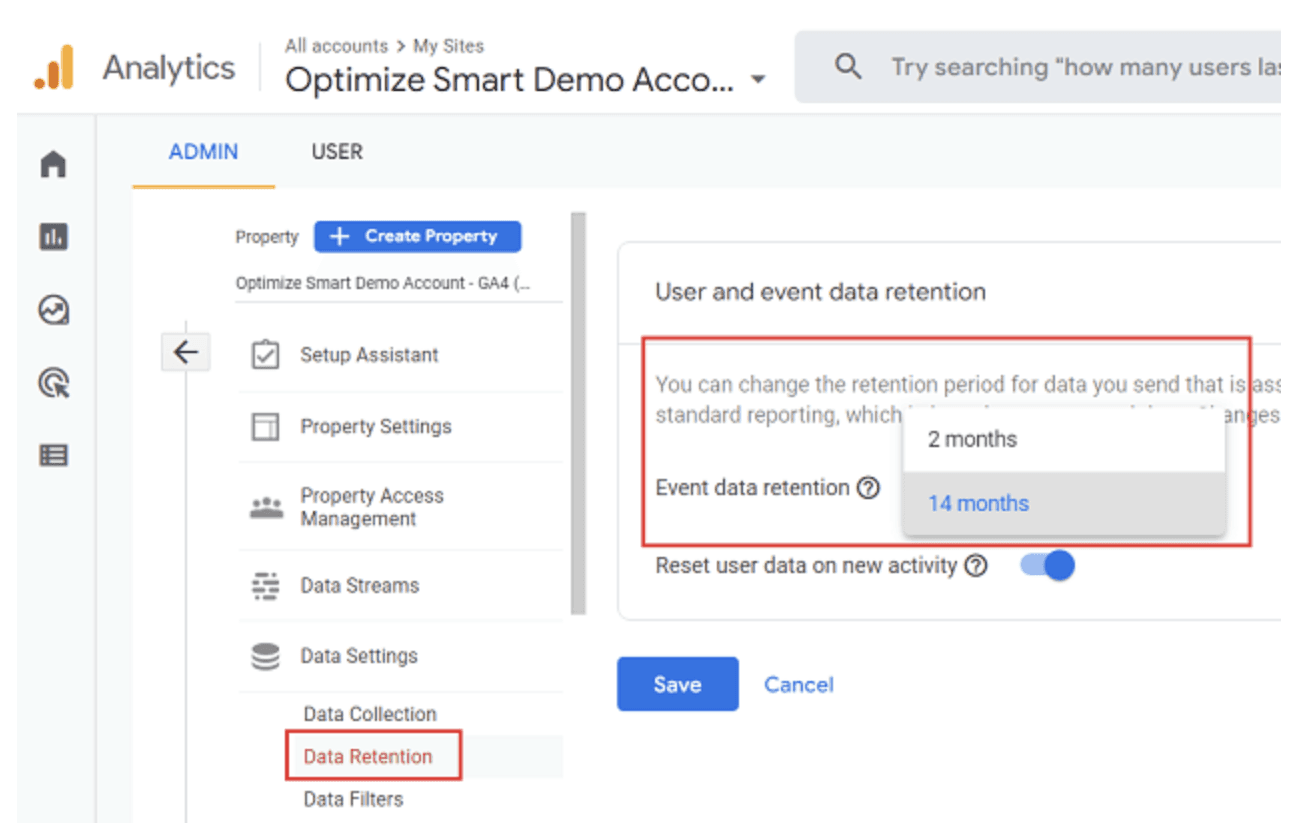
2. Access to Un-sampled Raw Events
Data sampling is a statistical method used to analyse a subset of data from a larger dataset to make inferences or predictions about the larger dataset.
Why is it done?
Well mainly to reduce the costs of compute as you can significantly reduce the volume of data being processed. Data sampling is also simplifies complex data sets making them more manageable and easier to analyse.
But Sampling has it's downsides too. Since it involves selecting only a portion of the dataset to analyse instead of examining every single data point, there's a risk of missing out on key data points; which subsequently results in a lack of granularity and could lead to less informed decision making.
GA4 Limitation: In Google Analytics, data sampling may occur when the number of events used to create a report, exploration, or request exceeds the quota limit for your property. The quota limit for event level queries is 10 million events for standard Google Analytics properties.
BigQuery Advantage: Provides access to un-sampled, raw event data, ensuring analyses are based on complete data sets.
What this means for you: Having access to every piece of data ensures your analyses are as accurate and reliable as possible, crucial for making informed decisions and understanding the full scope of user behaviour.

3. Cardinality
How often do you see your data grouped in the 'Other' row in GA4? That's when your data set has hit the Cardinality limit in GA4 for that particular table/dimension.
Cardinality refers to the number of unique values assigned to a dimension.
High cardinality refers to a situation where a column or a dataset has a large proportion of unique values. Imagine a database column that stores user IDs. Since each user ID is unique, this column would have high cardinality.
Low cardinality, on the other hand, describes a column or dataset with many repeated values and fewer unique ones. A simple example would be a column that records user gender in a dataset. With only a few possible values (e.g., Male, Female, Other), this column exhibits low cardinality.
GA4 Limitation: Google does not specify the cardinality limit for an individual dimension but it defines High-cardinality dimensions as dimensions with more than 500 unique values in one day. Which means reports with more than 500 unique values in a dimension run the risk of data being grouped into the 'Other Row'.
BigQuery Advantage: Big Query handles high-cardinality data without any limitations, ensuring detailed data points are preserved and reportable.
What this means for you: This level of detail enables you to perform nuanced analysis, segmenting your audience or behaviours with precision and uncovering insights that could be missed due to data aggregation in GA4.

4. Latency
Well we all know what latency is but Google calls this 'Data Freshness'. And here's how Google defines it - "Data freshness is how long it takes Google Analytics to collect and process an event from your property."
Which simply means the time taken for Google from the moment data is collected to the point it becomes available for analysis or reporting.
GA4 Limitation: Google Analytics allows for a data processing delay of 24-48 hours. Which means that any changes you make in your data collection structure will only show up in reporting post a 24-48 hour period. Quite frustrating isn't it?
BigQuery Advantage: When you sync GA4 to Big Query, you don't need to wait. Real time data captured in GA4, will flow directly into Big Query - sitting nicely for you to analyse it to your heart's desire.
What this means for you: The agility to adapt marketing efforts instantly in response to live data, optimising campaigns in real-time for maximum effectiveness.

5. Advanced data segmentation and analysis
Let's admit it, the analysis stage is the most exciting part of the data lifecycle journey. It's when you've gone through the tedious work of properly collecting and cleaning your data and it's now ready to be sliced and diced at your will.
But this is where GA4 lets you down. The primary challenge lies in GA4's user interface (UI), which can restrict the depth and flexibility of data analysis.
GA4 Limitation: GA4's user interface, for all its improvements, imposes limitations on your ability to get creative with your analyses. How often do you run into restrictions that don't allow certain combinations of dimensions and metrics to be mapped together? Quite often, we know.
Big Query Advantage : SQL. SQL is recognised as the industry standard for data analysis. It's a language specifically designed for querying and manipulating databases! And it's how you speak to your data in Big Query.
What this means for you: Using SQL in Big Query, you can bypass the limitations of GA4's UI and slice and dice your data for complex analyses that lead to awesome insights.

6. Cross Platform Data Integration
In today's digital landscape, user interactions with a business span multiple touch-points, from social media engagements and website visits to email communications and offline encounters. This has transformed user journeys into intricate webs of interactions, and consequently, data generated from these diverse touch-points is siloed across different platforms, such as CRM systems, social media analytics, and e-commerce platforms.
When analysing a user journey, it's then crucial for us to view and analyse data in a unified database.
GA4 Limitation: GA4 primarily focuses on web and app data, with limited capacity for integrating external data sources.
BigQuery Advantage: As a data warehouse, BigQuery excels in integrating data from multiple sources, for comprehensive analysis.
What this means for you: By consolidating data from various touch-points you gain access to a holistic view of customer interactions. And this enables you to identify patterns and correlations between different channels and user behaviours that were previously siloed.
7. Build Audiences For Ad Targeting
Segmenting users into audiences has been a core practice for marketers since the time marketing first began. And while we've come a far way when it comes to the level of sophistication in audience segmenting, there's still a long way to go.
Most marketers today lean on a heuristics based approach when it comes to building audiences. They rely on proxy events (signups, session duration, activations etc) which they hope to god are linked to conversion and high CLTV.
But as user journeys have grown more complex, this method starts to feel a bit like shooting in the dark. It's too simple for the labyrinthine paths users take today.
Enter the power of AI and ML.
GA4 Limitation: Allows for simplistic audience segmentation with event and property based filters.
Big Query Advantage: With access to historical data and multi-channel data integration, BigQuery enables machine learning models to be built on top for advanced analytics.
What this means for you: No more spray and pray. You can now increase your ROAS by leveraging AI to build Audiences that are most likely to convert or meet your CLTV threshold.
How do you build these AI models?
Well you don't need to. Toplyne will do it for you.
Toplyne is a data scientist for marketing teams. And what we do really well is leverage your first party data (wherever it may reside) to build custom AI audiences that are most likely to meet your business objectives.
We then sync these audiences back into your Ad platforms so you can optimise your Ad spend and increase your returns. Get the best bang for your buck as they say.
Interested to see how this works? See Toplyne in action here.
There's many more reasons why syncing GA4 to Big Query is a no brainer -
Unrestricted Reporting in Looker Studio: GA4’s integration with Looker Studio is bound by API request limits, potentially hampering in-depth analysis. BigQuery integration removes these barriers, providing unrestricted data access for advanced reporting.
Filter out incorrect data: Cleaning data is much easier when using SQL querying capabilities in Big Query. You can then easily modify incorrect data from your analysis and reports.
Solve for Scale: BigQuery's architecture is built for scalability, leveraging Google's cloud infrastructure to automatically adjust resources based on data volume and query complexity. Which means you don't need to worry about data management as your organisation grows.
Conclusion
Each of these advantages of integrating GA4 with BigQuery equips you with enhanced tools and capabilities for a more detailed, accurate, and timely understanding of your data, laying the groundwork for more effective, data-driven marketing strategies.
In December 2023, Google announced the next step in phasing out cookies globally. Google started testing Tracking Protection on Chrome, a new feature that limits cross-site tracking - signifying another big stride towards the end of the cookie-era. This comes on the back of Apple severely limiting advertiser access to IDFA, all indicating a general trend of third-party data's slow march to irrelevance.
As marketing teams scurry away from third party data to build audiences and targeting lists, first party data sources - the Google Analytics, Mixpanels, Amplitudes of the world are steadily moving to the center stage.
And for these marketing teams, Google Analytics is a treasure trove of data on user-behavior - both historical and current. As this data becomes a crucial cog in a marketer's segmentation arsenal, access to historical GA data is table-stakes.
And very few teams even own their own data for the simple reason that GA doesn't support storage of historical data.
Unless you've synced to Big Query.
Here are the top reasons why this is a smart move ➝
1. Data Retention
Data retention refers to how long a platform keeps the data before it's automatically deleted.
While Google Analytics 4 gives you the ability to set the amount of time before user-level and event-level data is automatically deleted from it's servers, there is a limit to the maximum length of time you can set for your data to be retained.
GA4 Limitation: In GA4, user-level and event-level data retention is limited to a maximum of 14 months. With the default data retention time set at 2 months. Please change this to 14 months if you haven't already.
BigQuery Advantage: BigQuery is a cloud based data warehouse and it's first job is to allow indefinite data retention, which means you can store your analytics data for as long as you need without the risk of deletion.
What this means for you: With timeless data retention in Big Query, you have access to all historical data, enabling thorough trend analysis over long term periods and the flexibility to conduct year-over-year comparisons without data loss. This depth of insight supports more informed strategic decisions.
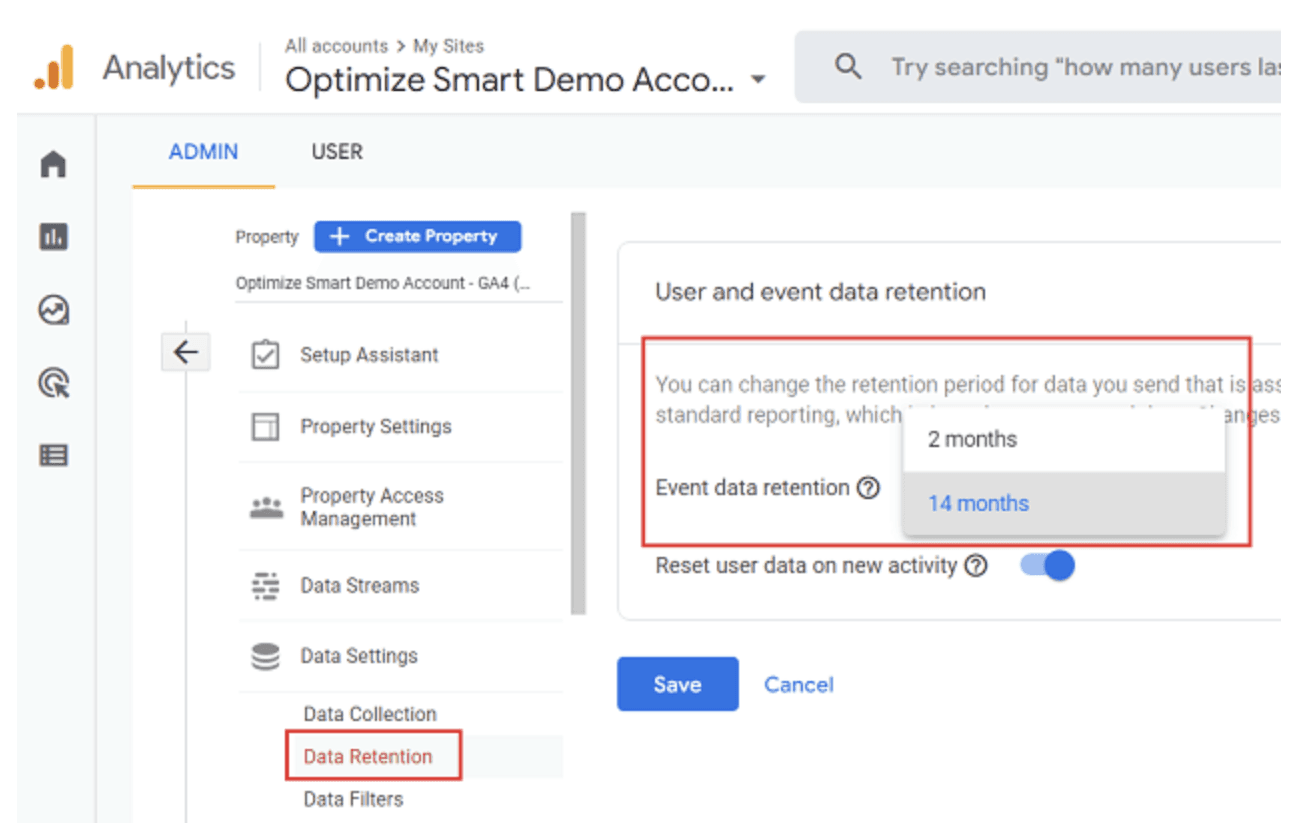
2. Access to Un-sampled Raw Events
Data sampling is a statistical method used to analyse a subset of data from a larger dataset to make inferences or predictions about the larger dataset.
Why is it done?
Well mainly to reduce the costs of compute as you can significantly reduce the volume of data being processed. Data sampling is also simplifies complex data sets making them more manageable and easier to analyse.
But Sampling has it's downsides too. Since it involves selecting only a portion of the dataset to analyse instead of examining every single data point, there's a risk of missing out on key data points; which subsequently results in a lack of granularity and could lead to less informed decision making.
GA4 Limitation: In Google Analytics, data sampling may occur when the number of events used to create a report, exploration, or request exceeds the quota limit for your property. The quota limit for event level queries is 10 million events for standard Google Analytics properties.
BigQuery Advantage: Provides access to un-sampled, raw event data, ensuring analyses are based on complete data sets.
What this means for you: Having access to every piece of data ensures your analyses are as accurate and reliable as possible, crucial for making informed decisions and understanding the full scope of user behaviour.

3. Cardinality
How often do you see your data grouped in the 'Other' row in GA4? That's when your data set has hit the Cardinality limit in GA4 for that particular table/dimension.
Cardinality refers to the number of unique values assigned to a dimension.
High cardinality refers to a situation where a column or a dataset has a large proportion of unique values. Imagine a database column that stores user IDs. Since each user ID is unique, this column would have high cardinality.
Low cardinality, on the other hand, describes a column or dataset with many repeated values and fewer unique ones. A simple example would be a column that records user gender in a dataset. With only a few possible values (e.g., Male, Female, Other), this column exhibits low cardinality.
GA4 Limitation: Google does not specify the cardinality limit for an individual dimension but it defines High-cardinality dimensions as dimensions with more than 500 unique values in one day. Which means reports with more than 500 unique values in a dimension run the risk of data being grouped into the 'Other Row'.
BigQuery Advantage: Big Query handles high-cardinality data without any limitations, ensuring detailed data points are preserved and reportable.
What this means for you: This level of detail enables you to perform nuanced analysis, segmenting your audience or behaviours with precision and uncovering insights that could be missed due to data aggregation in GA4.

4. Latency
Well we all know what latency is but Google calls this 'Data Freshness'. And here's how Google defines it - "Data freshness is how long it takes Google Analytics to collect and process an event from your property."
Which simply means the time taken for Google from the moment data is collected to the point it becomes available for analysis or reporting.
GA4 Limitation: Google Analytics allows for a data processing delay of 24-48 hours. Which means that any changes you make in your data collection structure will only show up in reporting post a 24-48 hour period. Quite frustrating isn't it?
BigQuery Advantage: When you sync GA4 to Big Query, you don't need to wait. Real time data captured in GA4, will flow directly into Big Query - sitting nicely for you to analyse it to your heart's desire.
What this means for you: The agility to adapt marketing efforts instantly in response to live data, optimising campaigns in real-time for maximum effectiveness.

5. Advanced data segmentation and analysis
Let's admit it, the analysis stage is the most exciting part of the data lifecycle journey. It's when you've gone through the tedious work of properly collecting and cleaning your data and it's now ready to be sliced and diced at your will.
But this is where GA4 lets you down. The primary challenge lies in GA4's user interface (UI), which can restrict the depth and flexibility of data analysis.
GA4 Limitation: GA4's user interface, for all its improvements, imposes limitations on your ability to get creative with your analyses. How often do you run into restrictions that don't allow certain combinations of dimensions and metrics to be mapped together? Quite often, we know.
Big Query Advantage : SQL. SQL is recognised as the industry standard for data analysis. It's a language specifically designed for querying and manipulating databases! And it's how you speak to your data in Big Query.
What this means for you: Using SQL in Big Query, you can bypass the limitations of GA4's UI and slice and dice your data for complex analyses that lead to awesome insights.

6. Cross Platform Data Integration
In today's digital landscape, user interactions with a business span multiple touch-points, from social media engagements and website visits to email communications and offline encounters. This has transformed user journeys into intricate webs of interactions, and consequently, data generated from these diverse touch-points is siloed across different platforms, such as CRM systems, social media analytics, and e-commerce platforms.
When analysing a user journey, it's then crucial for us to view and analyse data in a unified database.
GA4 Limitation: GA4 primarily focuses on web and app data, with limited capacity for integrating external data sources.
BigQuery Advantage: As a data warehouse, BigQuery excels in integrating data from multiple sources, for comprehensive analysis.
What this means for you: By consolidating data from various touch-points you gain access to a holistic view of customer interactions. And this enables you to identify patterns and correlations between different channels and user behaviours that were previously siloed.
7. Build Audiences For Ad Targeting
Segmenting users into audiences has been a core practice for marketers since the time marketing first began. And while we've come a far way when it comes to the level of sophistication in audience segmenting, there's still a long way to go.
Most marketers today lean on a heuristics based approach when it comes to building audiences. They rely on proxy events (signups, session duration, activations etc) which they hope to god are linked to conversion and high CLTV.
But as user journeys have grown more complex, this method starts to feel a bit like shooting in the dark. It's too simple for the labyrinthine paths users take today.
Enter the power of AI and ML.
GA4 Limitation: Allows for simplistic audience segmentation with event and property based filters.
Big Query Advantage: With access to historical data and multi-channel data integration, BigQuery enables machine learning models to be built on top for advanced analytics.
What this means for you: No more spray and pray. You can now increase your ROAS by leveraging AI to build Audiences that are most likely to convert or meet your CLTV threshold.
How do you build these AI models?
Well you don't need to. Toplyne will do it for you.
Toplyne is a data scientist for marketing teams. And what we do really well is leverage your first party data (wherever it may reside) to build custom AI audiences that are most likely to meet your business objectives.
We then sync these audiences back into your Ad platforms so you can optimise your Ad spend and increase your returns. Get the best bang for your buck as they say.
Interested to see how this works? See Toplyne in action here.
There's many more reasons why syncing GA4 to Big Query is a no brainer -
Unrestricted Reporting in Looker Studio: GA4’s integration with Looker Studio is bound by API request limits, potentially hampering in-depth analysis. BigQuery integration removes these barriers, providing unrestricted data access for advanced reporting.
Filter out incorrect data: Cleaning data is much easier when using SQL querying capabilities in Big Query. You can then easily modify incorrect data from your analysis and reports.
Solve for Scale: BigQuery's architecture is built for scalability, leveraging Google's cloud infrastructure to automatically adjust resources based on data volume and query complexity. Which means you don't need to worry about data management as your organisation grows.
Conclusion
Each of these advantages of integrating GA4 with BigQuery equips you with enhanced tools and capabilities for a more detailed, accurate, and timely understanding of your data, laying the groundwork for more effective, data-driven marketing strategies.
Related Articles




Generative AI: And the winner is...
Predicting who takes home the generative AI crown (and how)




Propensity Models in Sales: What You Need to Know
The only guide you'll need to understand what propensity models are in sales, the types of propensity models, their use cases, and how to implement them.




Lead Grading Essentials: The B2B Sales Guide
The only guide you'll need to understand lead grading essentials.
Copyright © Toplyne Labs PTE Ltd. 2024
Copyright © Toplyne Labs PTE Ltd. 2024
Copyright © Toplyne Labs PTE Ltd. 2024
Copyright © Toplyne Labs PTE Ltd. 2024