Predictive Lead Scoring (How It Works, Benefits, Drawbacks)
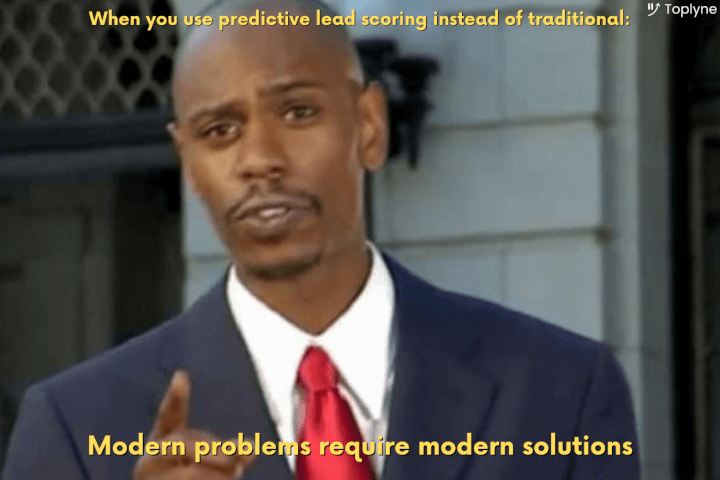
If your sales team is working at total capacity, chances are you're contacting anywhere from tens to thousands of leads every single day.
But in what order…?
What if we told you there’s a way you can refocus your sales strategy to reach out to high-intent leads first and then make your way down the ladder? 💡
This way, your sales team can make some real progress instead of…
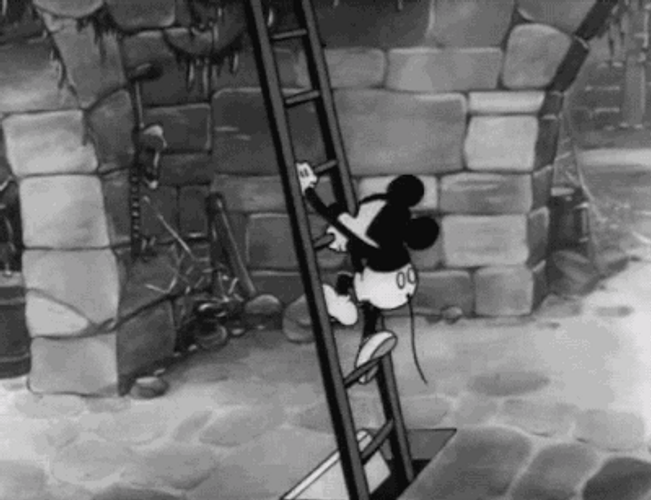
With predictive lead scoring, you can take the guesswork out of the entire process! It’s how your sales reps can reach out to high-quality leads and get them to convert quickly.
This article will explore the nitty-gritty of predictive lead scoring, the key customer data you need for it, how it works, and some of its benefits and drawbacks.
We’ll introduce you to a kick-ass tool that combines the power of predictive lead scoring AND go-to-market motions!🪜
Let’s get started!
What is Predictive Lead Scoring?
Predictive lead scoring is an algorithm-based machine learning model that uses all historical customer data to calculate a score for each lead.
Locked and loaded with all the necessary data, your reps can prioritize the right leads and save dozens of hours every sales cycle!
For instance, if Customer A has a score of 85 and Customer B has a score of 60, the sales rep should prioritize Customer A as they’re more likely to convert and become a loyal customer.
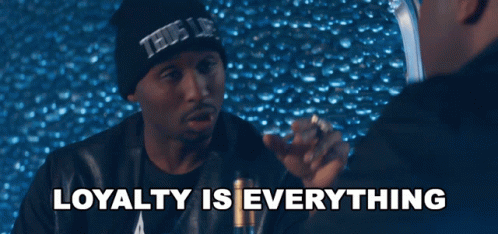
But why should you be loyal to predictive lead scoring?
Sales teams are increasingly adopting machine learning and artificial intelligence to automate processes and reduce CAC (Customer Acquisition Cost).
So if you want to keep up with these trends, predictive analytics can be the wind in your "Sales." ⛵
Ready to ride the wave 🌊 of high-powered growth?
Let’s dive deeper into what data you need to generate an accurate predictive score.
Key Customer Data You Need for Predictive Lead Scoring
Every great dish starts with the right ingredients.
For a fully functioning predictive scoring model, you need the data — freshly sourced from your user database, analytics, and CRM tools.
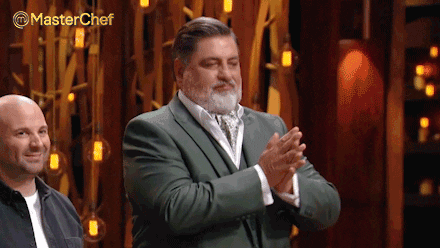
Here are some data points your lead scoring model needs to deliver to you, one qualified lead after another!📝
- Demographic Data: This is your primary customer data like their name, age, date of birth, and address.
- Purchase Data: This includes how often customers purchase your products and which ones they choose. It can help you forecast their buying patterns. 🧾
- Engagement Data: Customer engagement data refers to how much the customer engages with your brand through website views, link clicks, freemium downloads, etc.
- Company Information: Information such as which company or industry your potential customer works in can help you personalize product recommendations.
- Campaign Data: Understanding how your sales and marketing campaigns perform is crucial for understanding future customer behavior.
- Product-usage Data: Actions your users take within your product - the most impactful indicator of conversions.
Skip the recipe and get a ready-made pipeline of piping-hot qualified leads with Toplyne.
Or learn —
How Does Predictive Lead Scoring Work?
The critical difference between predictive lead scoring and other manual methods is that it can be automated. Its fast-paced, self-learning algorithm is the basis for predictive lead scoring’s speed and accuracy.
Take a look at these steps to automate a predictive lead scoring model:
- Step 1: Identify each data point and target attribute you want to measure. For instance, for your company, downloads might be an essential metric. These can be easily collected through your CRM (Customer Relationship Management) system.
- Step 2: After this, you need to specify a point value for each data point. The predictive lead scoring process then uses machine learning algorithms to create a model. This model can accurately assign customers’ a lead score based on their demographic and historical data.
These models can also help you identify shortcomings in your offerings and customer preferences, ensuring you don't lose out on a big sale.
- Step 3: Depending on your chosen attributes and requirements, you can use multiple approaches to generate an accurate lead score. The most common machine learning model is called Logistic Regression.
Feel like your brain is going through a regression?
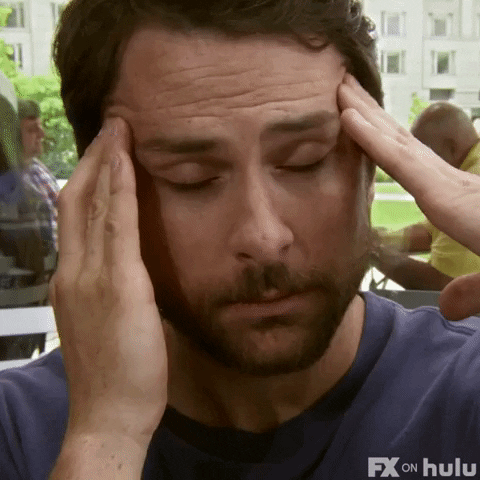
Logistic regression in big data analytics helps you develop a clear, specific value, where the max is typically 100. So, in this instance, the model gives a predictive lead score between 0 and 100 for each data point, which you would’ve specified in Step 2.
A higher predictive lead score (ideally 80+) means the prospect is more likely to convert to a paying customer, while those with lower scores (less than 50) can be dismissed as unqualified leads.
And that’s it!
The lead scoring software takes over the process, leaving your marketing and sales team free to focus only on lead conversion.
How does this process differ from manual or traditional lead scoring?
In the traditional lead scoring method, a marketer or sales rep uses demographic data to manually rank customers from most likely to least likely to buy.
That means specific data points (like a prospect visiting your product’s pricing page) might not even be counted, which could mean many leads are slipping through the cracks!
The horror! The horror!
To ensure this frightful situation doesn’t happen to you, we recommend using the predictive model!
Plus, a predictive lead scoring model also takes away the guesswork from attributing scores to leads.
Why does visiting the pricing page give a lead more points than visiting your blog?
Because historically, those leads have converted more often, and the predictive model knows that!
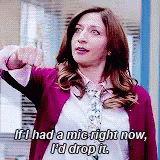
And your sales rep can’t always know how many times someone visited your pricing page. That’s the job best left for a predictive scoring tool.
Not convinced yet?
Just wait! 😉
Predictive Lead Scoring: Benefits and Drawbacks
While predictive lead scoring has many benefits, it also has some drawbacks. Let's explore them briefly:
A. Benefits
Here are three benefits of using a predictive scoring model:
1. Harmonize Your Sales Team and Marketing Team
With separate processes, tools, and KPIs, your marketing and sales teams may resemble estranged siblings rather than partners in crime.
For example, your marketing team might wonder why their lead generation and marketing efforts don't materialize into lead conversion.
Similarly, the sales team might want more leads to increase their chances of closing a lead.
Don’t hate the players. They need something to make them feel like they’re working towards the same objective.
With predictive analytics, both teams can understand the data and produce higher-quality leads.
Want more product-qualified leads? Spot them easily with Toplyne!
2. Reduce Human Error
To err is to be human. Sometimes we push a door that clearly says pull. 👀
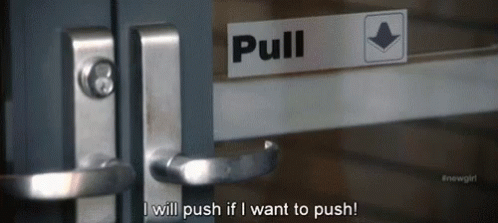
That’s why we need lead scoring software solutions.
A predictive scoring tool will drastically improve the error margin on your lead scoring process and deliver consistently good results.
It also eliminates the risk of personal biases affecting lead scores. A computer does what it’s been told and looks at all data ob-jec-tively 🤖.
3. Cut Down on Time and Costs Spent on Chasing Leads
Traditional lead scoring models require reps to evaluate each potential lead before chasing it.
As a result, your team could spend a lot of time chasing poor-quality or unqualified leads.
If time is money, traditional lead scoring is burning it. 🔥
On the contrary, predictive lead scoring saves time and money from the get-go.
No manually sorting through leads to score them. ✅
No more chasing bad leads to make a sale. ✅
What can you do with all that extra time and money?
You’re the boss. 🤷
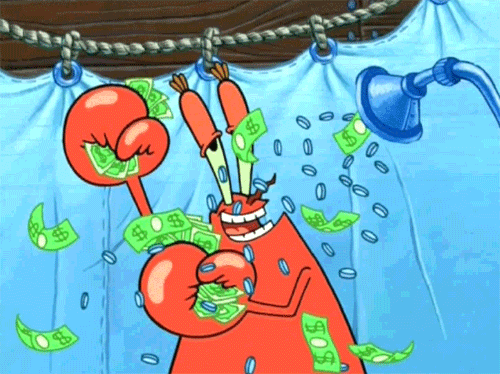
B. Potential Drawbacks
Here are some potential drawbacks of using a predictive lead scoring model:
1. Needs a Large Data Pool
One of the most significant limitations of a predictive model is that it needs a large dataset of customers.
You could end up spending hours gathering data about your customer’s firmographics, website engagement, email engagement, buying preferences, favorite color, pet’s name, etc. 😮💨
Want to skip the painful parts?
Use Toplyne to automatically process ALL your user behavior data to find out which lead will likely become a potential customer.
2. Most Predictive Models are Black Boxes
Sounds ominous?
That’s because it is. 💀
Predictive lead scoring models are like expensive gadgets. Shiny and smooth to look at, but full of mysteries.
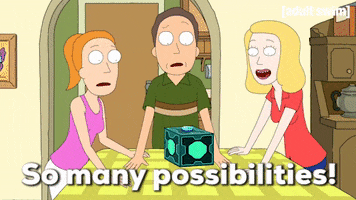
These black boxes also need at least 18 months' worth of historical data to predict future behavior, which basically rules them out for upstart companies.
And for companies who can afford them, the delay in on-ground results can deter sales teams from adopting them.
But with Toplyne, all these issues seem like NBD because you got the best lead scoring tool there is! Everything about Toplyne is as transparent as Casper, so you know specifically how each lead is scored.
3. Might Require Reps with a Technical Background
Companies hire salespeople for their persuasive skills and understanding of the market.
So it defeats the purpose if you have to retrain them to use predictive lead scoring tools like Google Tag Manager in Google Analytics!
Moreover, machine learning, big data, and artificial intelligence are daunting topics that only some reps and marketers understand.
So, it would be best if you had a straightforward tool to do the lead scoring while you sit back and reap the rewards.
Wish granted! 🧞
Toplyne: The Only Tool You Need to Get Qualified Leads!
All this talk of machine learning and logistical regression may have given you a headache.
Hey, don't worry. We got something that’ll give your brain a breather.
We’ve got just the right tool for you — Toplyne!
Toplyne helps SaaS companies identify the users and accounts (leads) that are most likely to convert to paying customers based on their previous interactions with your company.
How, you ask?
First, Toplyne integrates with your product usage data sources to assemble an army of high-intent users. This is your fully formed, product qualified leads pipeline.
Next, you can target these quality leads with the right combination of in-app nudges and sales assist motions to make your win rates soar!
Finally, keep cycling these leads within your sales suite to identify nuanced opportunities for expansion revenue!
The best part?
No training. No setup cost. No change management at all!
Here’s how companies like Canva and Vercel generate sales pipeline from their self-serve funnel using Toplyne:
- Step 1/7: Create monetization playbooks to surface conversion and expansion opportunities (leads most likely to convert to paying customers, and teams most likely to grow into larger teams)
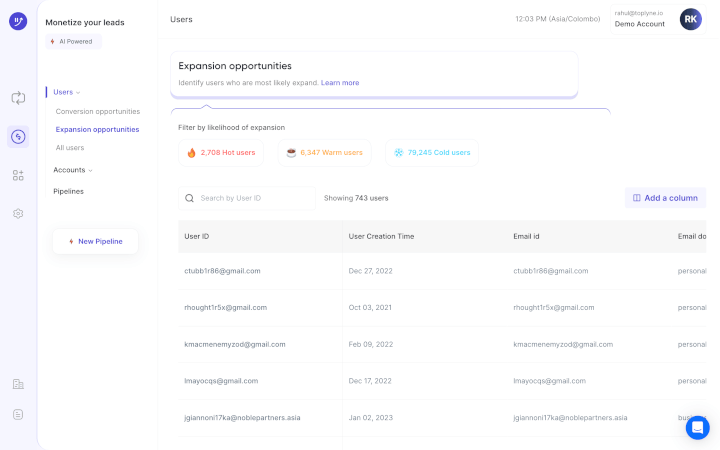
- Step 2/7: Choose the right leads to target – users (individual users) or accounts (a group of users with an organization).
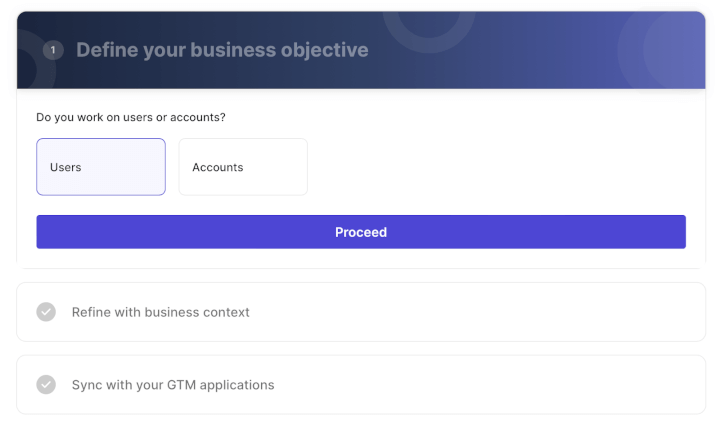
- Step 3/7: Select the frequency at which you would want leads synced in your GTM apps.
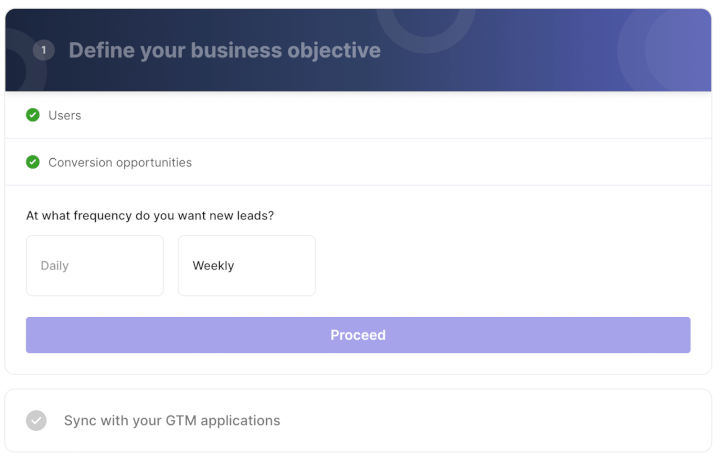
- Step 4/7: Define how many leads you want by either the number of leads or your expected win rate, depending on your sales capacity and GTM strategy.
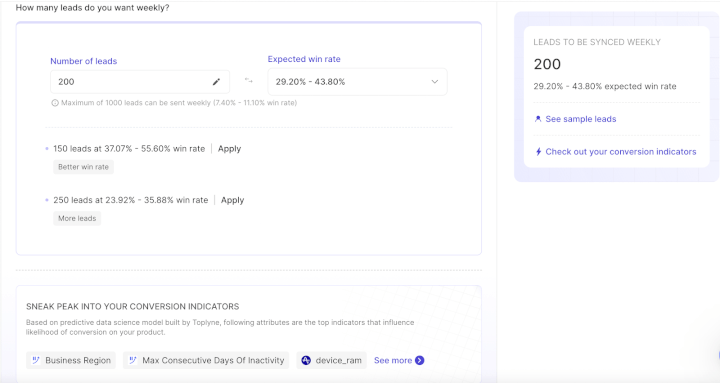
- Step 5/7: Build custom segments - Build custom segments based on And/Or logic at the deepest level of sub-properties within your product analytics.
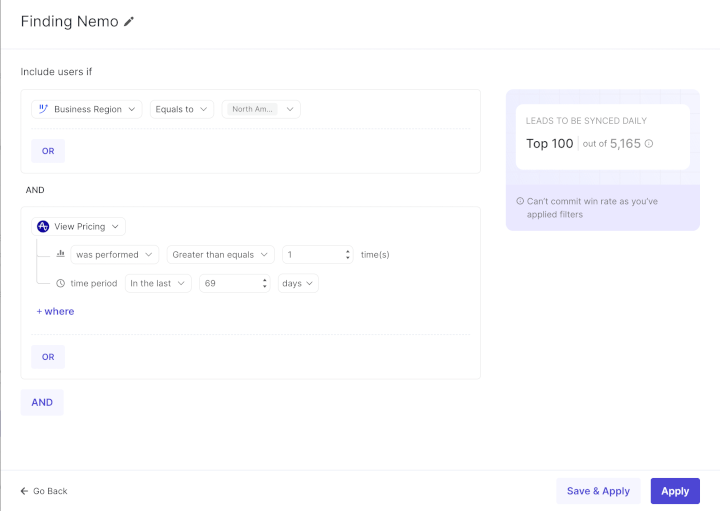
- Step 6/7: Validate your GTM strategy - Hold back some users as a control group to test your GTM strategy.
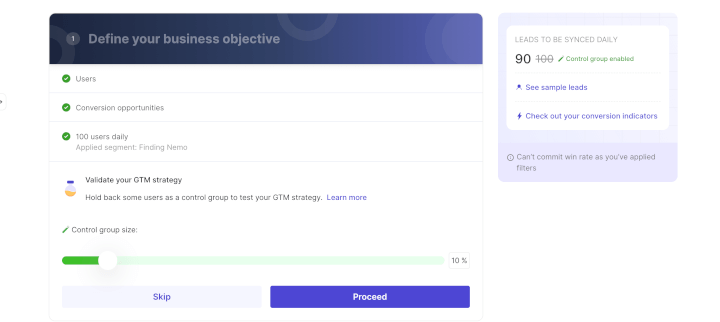
- Step 7/7: Sync your product qualified pipeline into your GTM destinations - CRMs, sales & marketing execution tools, and customer engagement platforms.
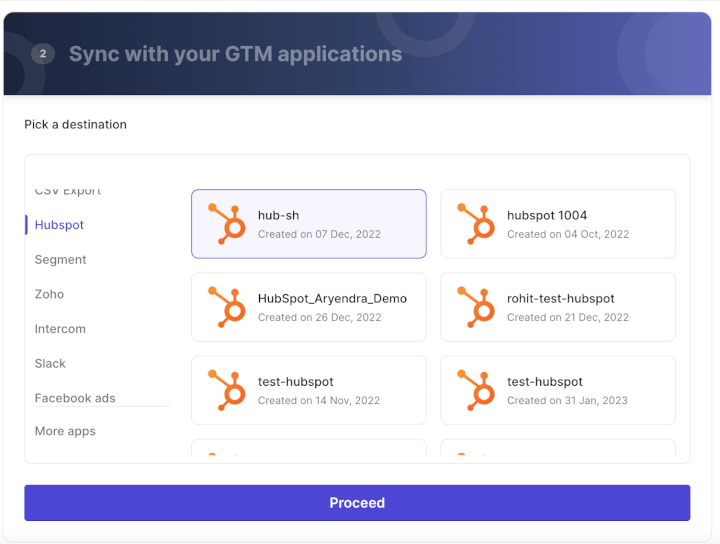
Like you’d say to your leads: don’t miss out on this opportunity of a lifetime! 😉
Step Up Beyond Predictive Lead Scoring with Toplyne!
Predictive lead scoring is a given for companies with hundreds or thousands of inbound leads. But on its own, it can be quite cumbersome and confusing.
After all, how do you know exactly what your leads want you to do?
You’re not a mind reader!
With Toplyne, you can connect your existing user analytics and sales workflow tools to hit your win rate and Net Retention Revenue (NRR) targets!
It’s time to pen your own success story.
So what are you waiting for?
Sign up for Toplyne's free trial today to easily predict your future growth! 😉
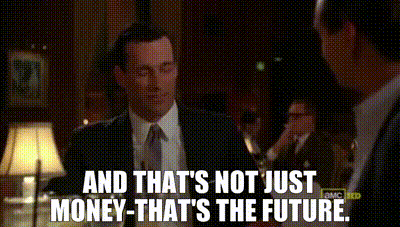






.svg)






